Multiscale Modeling of Porous Materials Enhanced by Deep Neural Networks
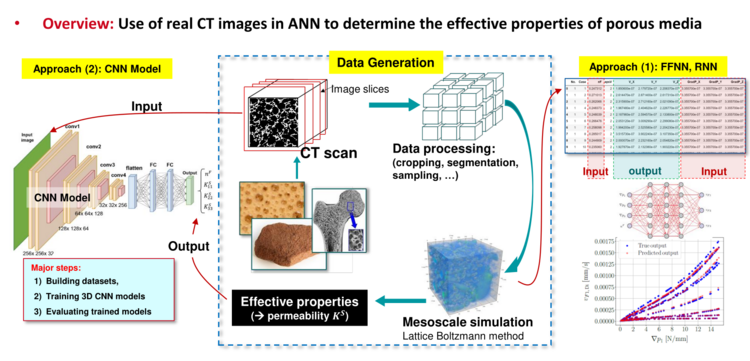
Leitung: | Yousef Heider |
Team: | Fadi Aldakheel |
Jahr: | 2024 |
Within the multiscale simulation of multiphase porous media, this research project focuses on Artificial Neural Network (ANN) applications to generate material models or predict macroscopic characteristics via supervised machine learning (ML). While feed-forward regression neural networks (FFNN) can be applied for capturing the history-independent material parameters, such as the permeability tensor, the recurrent neural networks (RNN) or the 1D Convolutional Neural Networks (CNN) can be used to capture history-dependent responses, such as the retention model [1,2].
The database used in the current supervised machine learning (ML) relies on the lower scale one- and two-phase lattice Boltzmann (LB) simulations, applied to deformable and anisotropic representative volume elements (RVEs) of the porous materials (Bentheimer networks). A special focus is on the anisotropy and deformation dependency of the intrinsic permeability tensor.
Alternative to the feed-forward regression model that relied entirely on the LB outputs, we can utilize Convolutional Neural Networks (CNN) to predict the macroscopic anisotropic permeability tensor at different deformation states [3]. The inputs for the CNN model consist of binarized CT images of real microgeometry, whereas the output is the symmetric 2nd-order permeability tensor. Thus, the CNN approach can generate a universal permeability model that relies on a limited number of CT images of the real microgeometry to capture the flow in deformable and anisotropic porous materials.
References
- Heider, Y; Suh H.S.; Sun W. (2021): An offline multi-scale unsaturated poromechanics model enabled by self-designed/self-improved neural networks. Int J Numer Anal Methods;1–26. https://doi.org/10.1002/nag.3196
- Chaaban M, Heider Y, Sun W, Markert B. A machine-learning supported multi-scale LBM-TPM model of unsaturated, anisotropic, and deformable porous materials. Int J Numer Anal Methods. 2024; 48: 889–910. https://doi.org/10.1002/nag.3668
- Heider, Y; Aldakheel F.; Ehlers W. (2024): CNN-powered micro- to macro-scale flow modeling in deformable porous media. Meccanica; (under review)